Retrieval-Augmented Generation (RAG)
Transform AI with Teneo and RAG
Create accurate, effective, and cost-optimized LLMs with Teneo RAG, which solves the lack of visibility, monitoring, control, and insights for live RAG deployments built in AI Studio, PromptFlow, or LangChain.
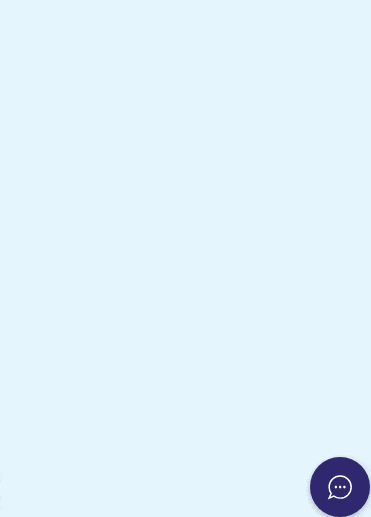
Teneo RAG in Action
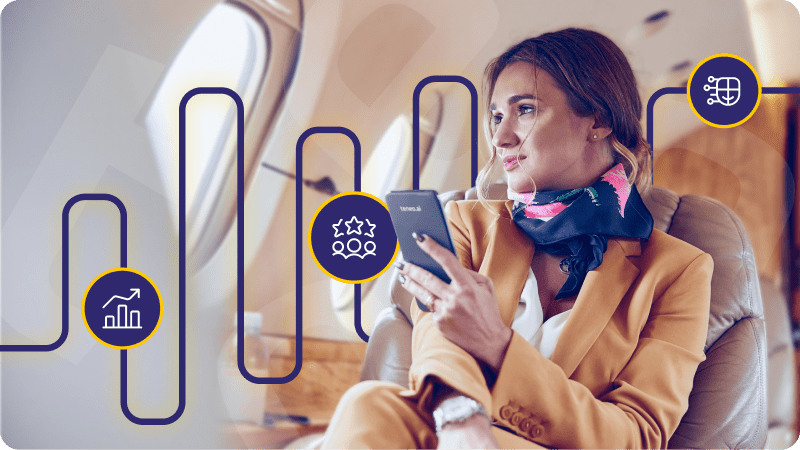
6 Challenges with RAG
While RAG offers promising AI capabilities, transitioning from a proof of concept (POC) to a live environment presents challenges:
Monitoring and Control
Effective monitoring and control mechanisms are essential to manage RAG's output, a key challenge in maintaining consistent quality in live scenarios.
Limited Visibility and User Feedback Integration
The lack the capability to fully see and understand user interactions, hindering the ability to control responses and integrate user feedback effectively.
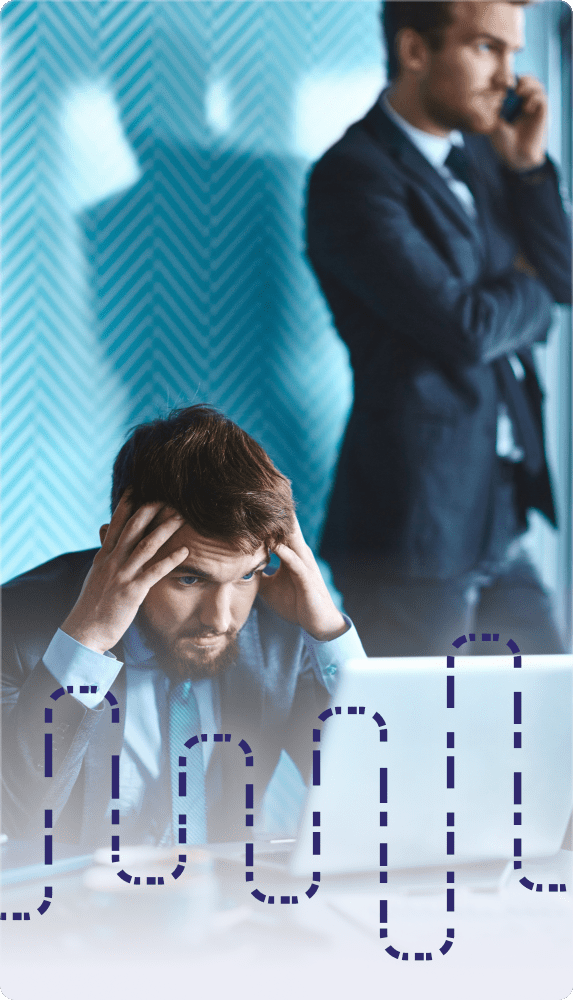
Maintaining Relevance and Accuracy
Continuous updates and maintenance are necessary for RAG to stay relevant and accurate, a task that grows increasingly complex in live environments.
Complex Integration
Integrating RAG into existing systems comes with high cost of technical labour and often becomes a hurdle in moving from POC to full-scale deployment.
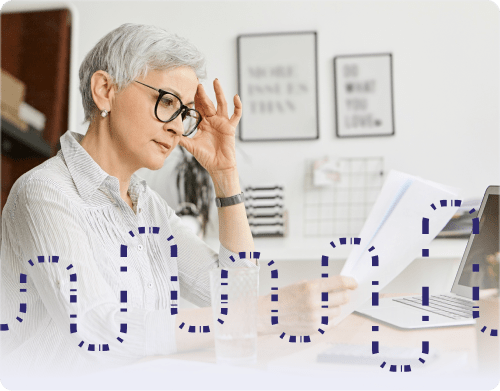
Scalability Issues
Scaling RAG from a controlled POC environment to handle diverse, real-world data and interactions is challenging in performance and reliability.
Performance Optimization
Ensuring RAG operates with optimal speed and accuracy across various business scenarios is a significant challenge during the scaling process.
Introducing Teneo Copilot
Teneo Copilot allows you to use any LLM to generate entries into your Teneo solution. Effortlessly create new entries and responses using Copilot's user-friendly interface, speeding up your workflow and enhancing your development skills.
Generate Classes
Generate Responses
Create Entities
Add Your Own LLM
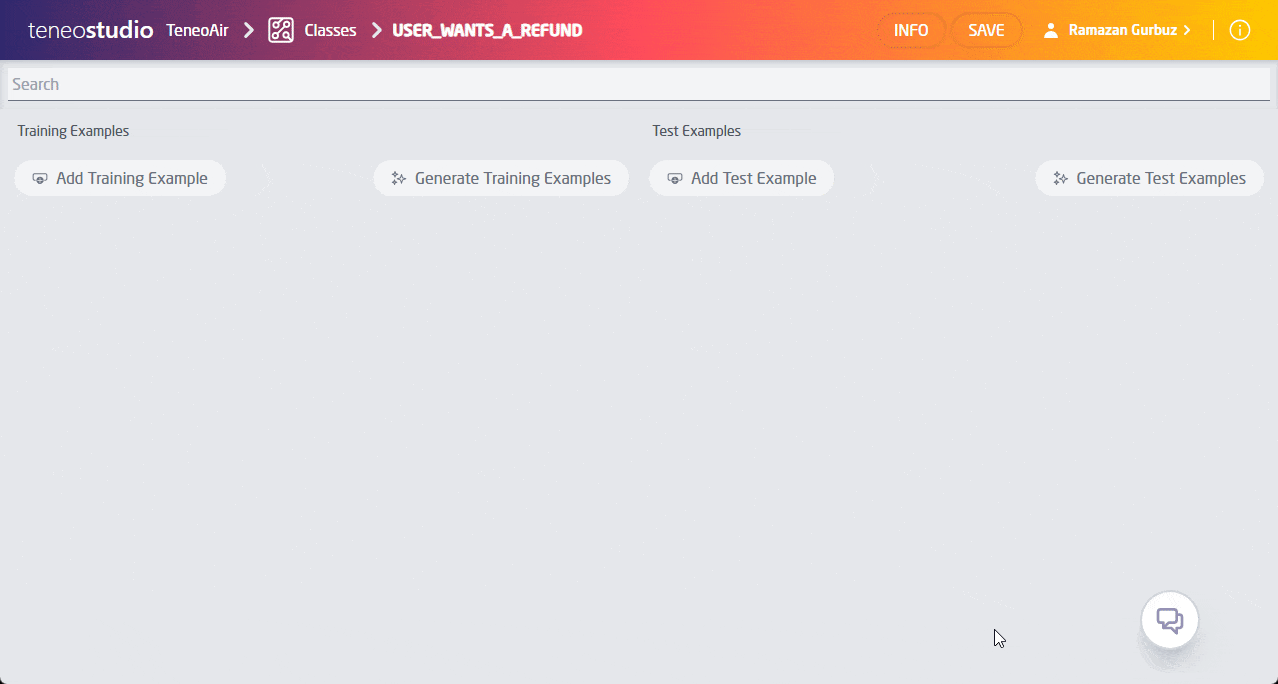
Why Teneo for RAG
With Teneo RAG your LLM will be accurate, effective and cost optimized.
Discover how Teneo makes RAG more efficient, reliable, and insightful for your business needs:
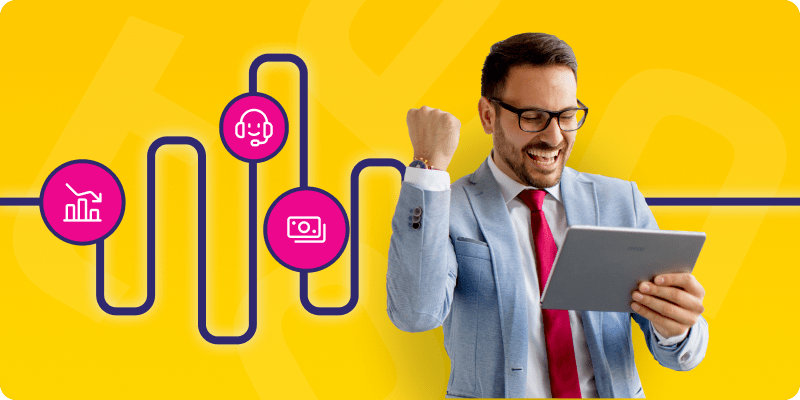
98% Cost Reduction
Teneo´s RAG is based on FrugalGPT and increase accuracy with prompt tuning.
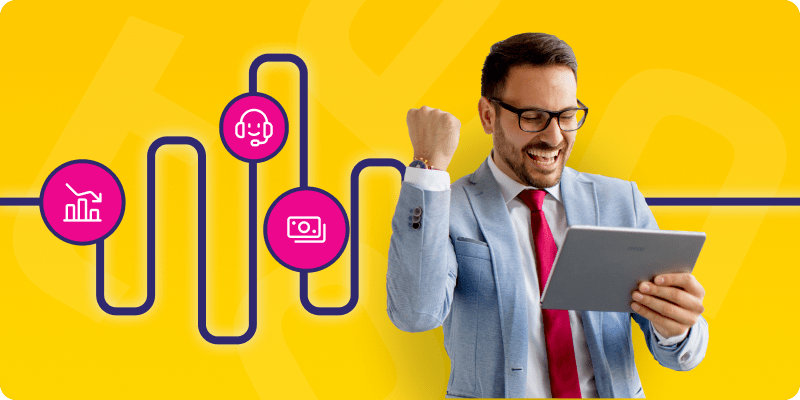
Monitor RAG Behavior
Teneo's monitoring tools allow businesses to understand and validate RAG's responses, ensuring AI interactions are aligned with business goals.
Control AI Responses
Adjust and refine RAG's outputs with Teneo's control features, ensuring accuracy and relevance in every interaction. Teneo cover the areas that RAG misses.
Listening to User Interactions
Teneo analyzes user interactions with RAG, providing insights to tailor AI responses to your audience's needs. Teneo defines which flow to call, extract info and orchestrates.
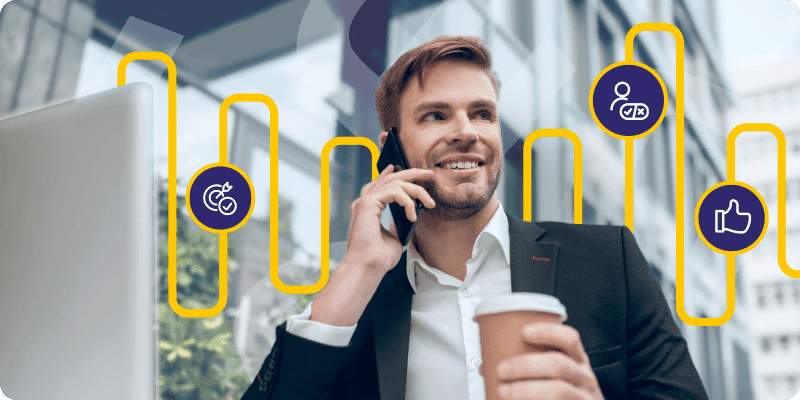
Benefits of Teneo RAG
Teneo enhances RAG's efficiency, ensuring faster and more effective AI operations.
Gain deep insights into user preferences and behavior with Teneo's advanced analytics.
Customize RAG's functionalities to suit specific business requirements and scenarios with Teneo's flexible tools.