Exploring the Potential of a RAG Pipeline
The development of robust and intelligent question-and-answer bots (QnA bots) is crucial for enhancing user experience and providing timely, accurate information. One of the cutting-edge technologies driving this advancement is the Retrieval-Augmented Generation (RAG) architecture. This approach combines the best of retrieval-based and generative systems to deliver high-quality answers that are both relevant and contextually rich.
What is RAG?
One question we need to identify is, what is a RAG. RAG voice chatbots integrate the retrieval of relevant documents into the generative process of creating responses. Essentially, when a query is received, the RAG pipeline first retrieves pieces of information (documents, snippets, etc.) that are likely to contain the answer and then uses a Generative AI model to synthesize and refine these into a coherent response. This methodology helps with improving the accuracy of the responses and significantly enhances their relevance by grounding them in verified information.
Choosing the Right Search method for RAG pipeline
The effectiveness of a RAG pipeline largely depends on its ability to search and retrieve the most relevant documents quickly. Therefore, different search methods can be employed to optimize this retrieval process and each has its own strengths. Let’s explore three innovative search options: Hybrid Search, Semantic Ranker, Vector Search, and HYDE Search, to understand their unique capabilities and ideal application scenarios.
Search Option 1: Hybrid Search
Hybrid Search merges traditional keyword-based search techniques with advanced natural language processing (NLP) capabilities. This approach is ideal for straightforward queries, where it can rapidly sift through data to find relevant information. Hybrid Search is particularly useful in environments like:
- FAQ systems and knowledge bases
- Product catalogs and e-commerce platforms
- Basic customer support interactions
Search Option 2: Semantic Ranker
Semantic Ranker delves deeper, analyzing the context and intent behind user questions. For example, it utilizes sophisticated NLP techniques to discern the relationships within text, offering answers that more closely align with the user’s intended meaning.
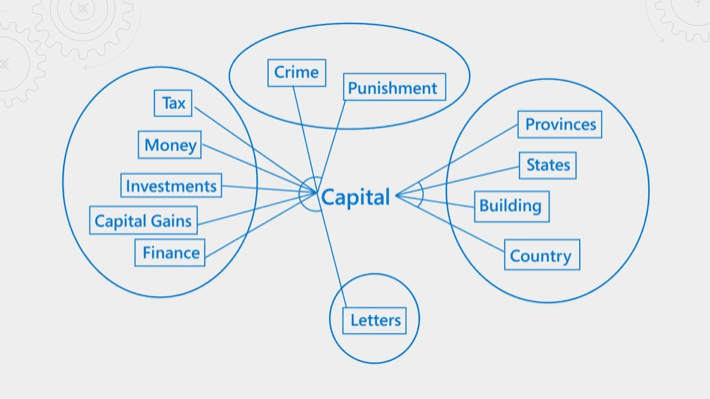
This is particularly effective for:
- Handling complex or nuanced inquiries
- Ensuring context is considered to deliver precise responses
- Catering to domain-specific requirements
Search Option 3: HYDE Search
HyDE (Hypothetical Document Embeddings) Search employs deep learning to refine query understanding. By constructing layered representations of words and phrases, this module captures linguistic subtleties, delivering highly accurate and context-sensitive results.
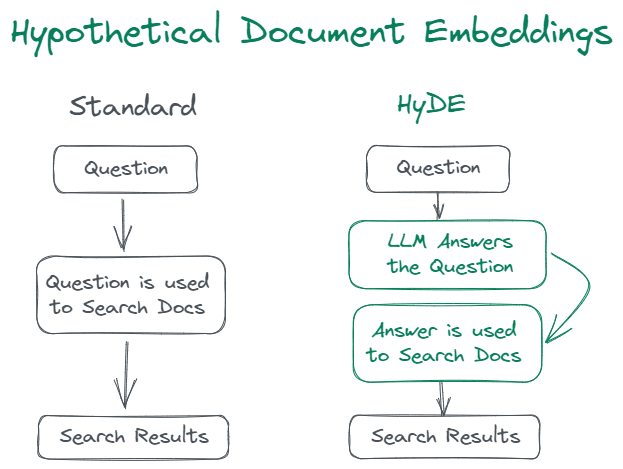
HYDE Search is most beneficial in situations where:
- Colloquial language or informal speech patterns are used
- Queries contain ambiguous or uncertain elements
- Critical decisions depend on the accuracy of the provided information
Search Option 4: Vector Search
Vector Search utilizes advanced algorithms to transform textual data into vector space, allowing for highly accurate semantic matching based on the content’s meaning rather than just keywords. This addition enhances your voice chatbots capability to handle even more complex queries with greater precision.
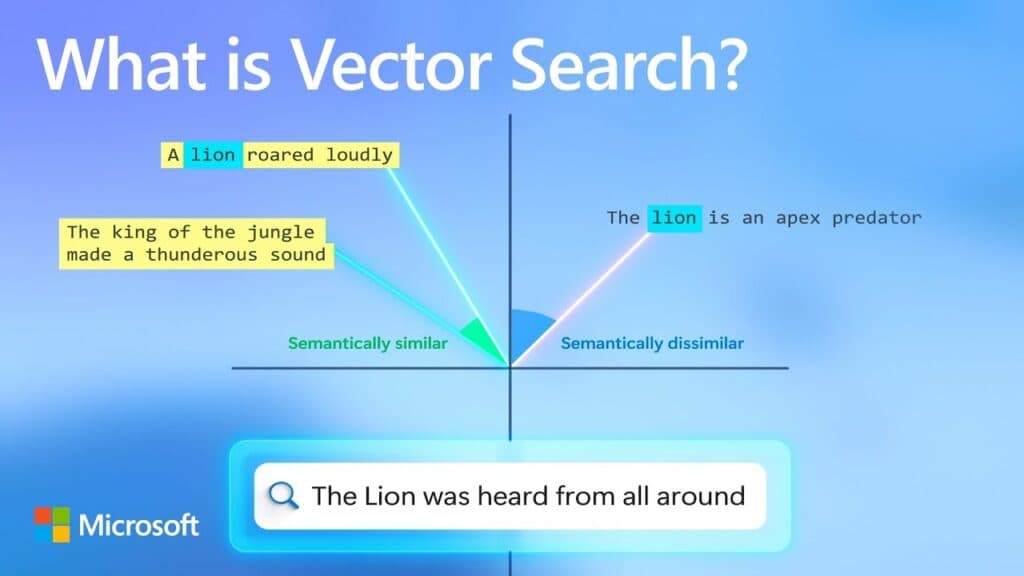
Selecting the Right Search Method for Your RAG voice chatbot
Choosing the appropriate search module is key to optimizing your RAG bot’s performance. Here’s a quick guide:
- For straightforward QnA: Hybrid Search handles basic queries efficiently, ideal for product details, FAQs, and simple support needs.
- For complex questions: Vector Search is recommended for detailed or context-rich queries, like those in specialized knowledge bases.
- For conversational dynamics: HYDE Search excels with informal dialogues and slang, perfect for interactive voice chatbots and voice interfaces.
Harness the Full Power of RAG Bots
By integrating these advanced modules, your RAG pipeline can adapt to a variety of scenarios, providing tailored and insightful responses. Whether you’re developing a support voice chatbot, an information-rich search engine, or an engaging conversational interface, our technology is designed to meet your needs.
Using Teneo for Building RAG Bots
Teneo is the ideal platform for developing RAG pipeline, supporting a full range of search methods including Hybrid Search, Vector Search, Semantic ranking, and HYDE Search. Furthermore, its robust framework is designed for seamless integration of these technologies, enabling the creation of precise and context-aware bots across various applications.
The platform streamlines the development process, making it straightforward to define project needs, configure search modules, and train bots with relevant data. I addition, Teneo’s intuitive interface and comprehensive capabilities allows you to build your RAG bots in 3 steps, thanks to its Generative QnA Template. In addition, Teneo helps you to:
Launch Your RAG pipeline Initiative Today!
Eager to enhance your QnA capabilities? Then, reach out to us to discover how RAG voice chatbot can transform your conversational interfaces. Our expert team is ready to help you design and implement a tailored solution that aligns with your strategic goals. Explore the future of your customer service with RAG chatbots today!